Our workshop on neuromorphic computing closed yesterday evening. We had a great line up of speakers from a diverse range of platforms (electronic, magnetic, optical, mechanical etc), which led to lively discussions and a fantastic atmosphere. The field is moving very fast, with many exciting advances in areas like novel training procedures for large-scale neuromorphic physical learning machines. Test applications by now include even large-language models. Maybe in a few years we will see those physical learning machines replacing digital neural networks in areas where energy-efficiency is key or sensors need to be tightly integrated from the start or ultralow latency is crucial. We plan to continue this workshop in 2 years.
Here is the workshop photo, taken on the stairwell of the Max Planck Institute for the Science of Light (Erlangen, Germany).
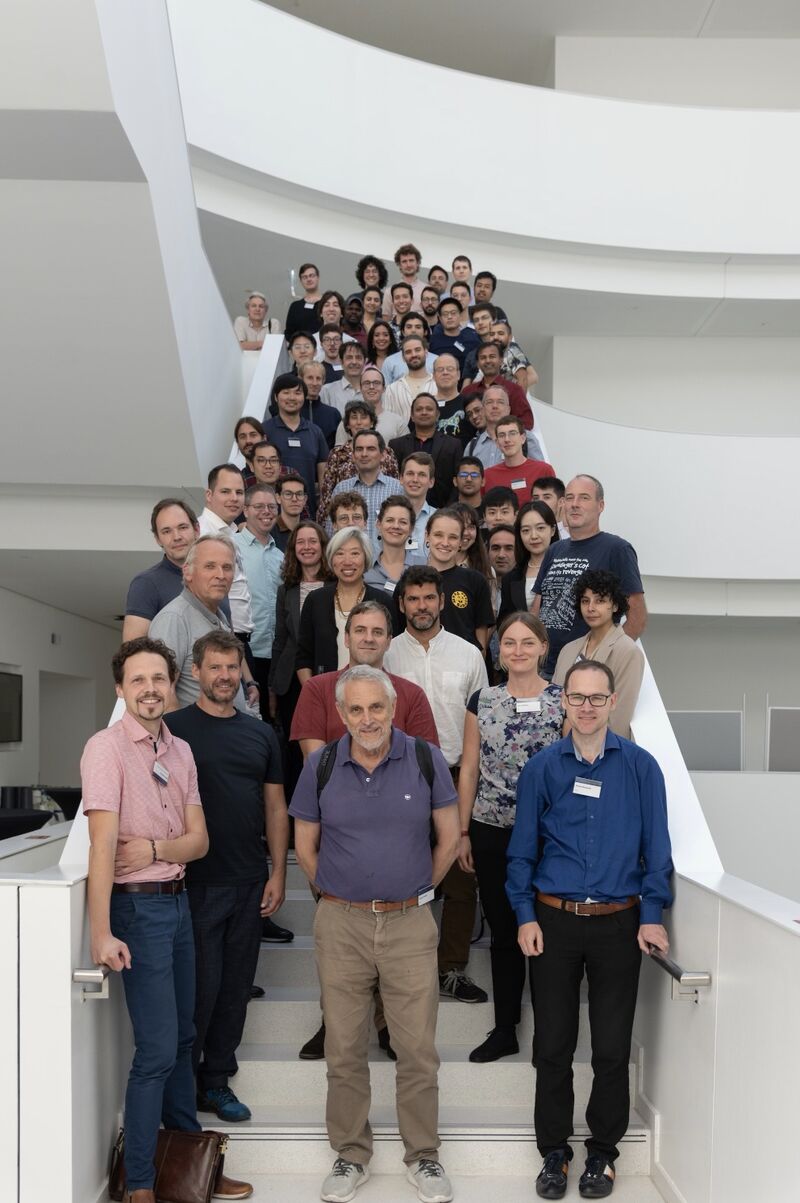
The Workshop on Frontiers of Neuromorphic Computing is taking place at the Max Planck Institute for the Science of Light in Erlangen 5-7 September 2023.
The workshop schedule is now available under the programme tab!
Registration deadline extended until 1 August!
(Registration for posters or attendance only will still be possible until 15 August.)
We have reserved a contingent of rooms for participants until 9 July. Please check the accommodation tab for details.
Frontiers of Neuromorphic Computing
The recent explosion of deep learning applications imposes an urgent need for new energy-efficient alternative neuromorphic hardware concepts running at high speeds and relying on a high degree of parallelism. In this workshop, we will explore this rapidly developing area of (classical) neuromorphic computing over a range of scalable platforms, both on a theoretical and experimental level. These platforms include systems in the domains of optics, integrated photonics, spin systems, semi- and superconducting systems, soft matter, and others. In addition, new physical learning approaches will be discussed.
Confirmed invited speakers
Wolfram Pernice (Heidelberg)
Julie Grollier (CNRF Thales):
Training physical systems with equilibrium propagation
Menachem (Nachi) Stern (U Penn):
Physical learning of energy-efficient solutions
Alexander Lvovsky (Oxford):
All-optical training of a neural network
Benjamin Scellier (Rain)
Alexander Khajetoorians (Radboud University):
Using model atomic spin systems to learn about in materia computing
Claudio Conti (Rome):
Theory and experiments in neuromorphic computing with classical waves
Sonia Buckley (NIST):
A general approach to fast online training on real neuromorphic systems
Daniel Brunner (CNRS, FEMTO-ST)
Abu Sebastian (IBM):
The IBM HERMES project chip: the most advanced analog in-memory computing chip based on memristive devices
Firooz Aflatouni (U Penn):
Integrated photonic deep networks for image classification
Tatsuhiro Onodera (Cornell):
Deep physical neural networks trained with in-situ backpropagation
Demetri Psaltis (EPFL)
Sylvain Gigan (Paris):
Exploiting light scattering for optical computing
Johannes Schemmel (Heidelberg):
Novel concepts for fast analog neuromorphic computing
Gyorgy Csaba (Budapest):
Training dynamical systems to do computing
Darius Bunander (lightmatter):
Accelerating AI through photonic computing and communication
Andrea Liu (U Penn)
Format
The in-person workshop will start on 5 September at 9 am and end on 7 September at approximately 5:30 pm. There will be invited talks, contributed talks, a poster session and a panel discussion. On Wednesday, we will organise a conference dinner which is included in the registration fee.